quentin dercon
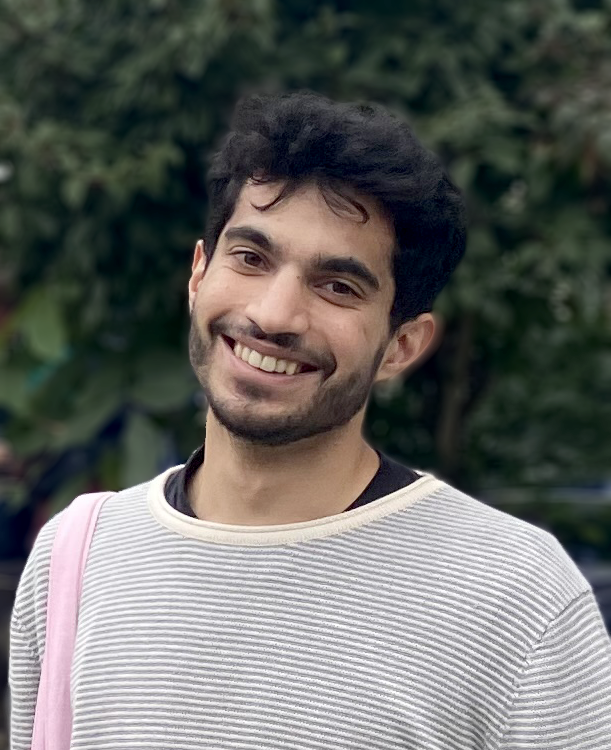
Hi there! My name is Quentin, and I’m a PhD student studying mental health science, based in the Applied Computational Psychiatry lab at UCL.
My current research interests include:
- how treatments for mental ill-health alter learning and other cognitive processes
- how we can formalise the mechanisms of individual components of psychological and pharmacological interventions
- how mechanistic insights can be used to predict responses to treatments and personalise clinical decision-making
Feel free to follow me on X to keep up to date with my work, or get in touch directly via the email icon at the bottom of the page.
selected publications
- Quentin Dercon†, Sara Z. Mehrhof†, Timothy R. Sandhu, Caitlin Hitchcock, Rebecca P. Lawson, Diego A. Pizzagalli, Tim Dalgleish, and Camilla L. Nord
Psychological therapy is an effective treatment for many mental health disorders, but its therapeutic mechanisms are relatively unclear. We investigated whether therapy may affect aspects of learning from rewards and losses, by comparing the performance on a learning task of participants who were asked to try to ’distance’ themselves from task feedback, to that of participants not engaging in so-called ’cognitive distancing’.
Participants engaging in cognitive distancing performed slightly better on the task. Using computational modelling, we found evidence that distancing led to choosing more driven by differences in expected values (i.e., the expected future return of a given option), and adaptive changes in how losses were used to compute and update these expected values. Our results indicate that distancing may improve symptoms of mental health disorders by promoting more effective engagement with negative information.
All analyses were implemented in a custom R package, pstpipeline, and each step is laid out in accompanying Jupyter notebooks alongside methodological motivations.
Background: Cognitive distancing is an emotion regulation strategy commonly used in psychological treatment of various mental health disorders, but its therapeutic mechanisms are unknown.
Methods: 935 participants completed an online reinforcement learning task involving choices between pairs of symbols with differing reward contingencies. Half (49.1%) of the sample was randomised to a cognitive self-distancing intervention and were trained to regulate or ‘take a step back’ from their emotional response to feedback throughout. Established computational (Q-learning) models were then fit to individuals’ choices to derive reinforcement learning parameters capturing clarity of choice values (inverse temperature) and their sensitivity to positive and negative feedback (learning rates).
Results: Cognitive distancing improved task performance, including when participants were later tested on novel combinations of symbols without feedback. Group differences in computational model-derived parameters revealed that cognitive distancing resulted in clearer representations of option values (estimated 0.17 higher inverse temperatures). Simultaneously, distancing caused increased sensitivity to negative feedback (estimated 19% higher loss learning rates). Exploratory analyses suggested this resulted from an evolving shift in strategy by distanced participants: initially, choices were more determined by expected value differences between symbols, but as the task progressed, they became more sensitive to negative feedback, with evidence for a difference strongest by the end of training.
Conclusions: Adaptive effects on the computations that underlie learning from reward and loss may explain the therapeutic benefits of cognitive distancing. Over time and with practice, cognitive distancing may improve symptoms of mental health disorders by promoting more effective engagement with negative information.
- Maxime Taquet, Quentin Dercon, Sierra Luciano, John R. Geddes, Masud Husain, and Paul J. Harrison
Background: Long-COVID refers to a variety of symptoms affecting different organs reported by people following Coronavirus Disease 2019 (COVID-19) infection. To date, there have been no robust estimates of the incidence and co-occurrence of long-COVID features, their relationship to age, sex, or severity of infection, and the extent to which they are specific to COVID-19. The aim of this study is to address these issues.
Methods and Findings: We conducted a retrospective cohort study based on linked electronic health records (EHRs) data from 81 million patients including 273,618 COVID-19 survivors. The incidence and co-occurrence within 6 months and in the 3 to 6 months after COVID-19 diagnosis were calculated for 9 core features of long-COVID (breathing difficulties/breathlessness, fatigue/malaise, chest/throat pain, headache, abdominal symptoms, myalgia, other pain, cognitive symptoms, and anxiety/depression). Their co-occurrence network was also analyzed. Comparison with a propensity score-matched cohort of patients diagnosed with influenza during the same time period was achieved using Kaplan-Meier analysis and the Cox proportional hazard model. The incidence of atopic dermatitis was used as a negative control. Among COVID-19 survivors (mean [SD] age: 46.3 [19.8], 55.6% female), 57.00% had one or more long-COVID feature recorded during the whole 6-month period (i.e., including the acute phase), and 36.55% between 3 and 6 months. The incidence of each feature was: abnormal breathing (18.71% in the 1- to 180-day period; 7.94% in the 90- to180-day period), fatigue/malaise (12.82%; 5.87%), chest/throat pain (12.60%; 5.71%), headache (8.67%; 4.63%), other pain (11.60%; 7.19%), abdominal symptoms (15.58%; 8.29%), myalgia (3.24%; 1.54%), cognitive symptoms (7.88%; 3.95%), and anxiety/depression (22.82%; 15.49%). All 9 features were more frequently reported after COVID-19 than after influenza (with an overall excess incidence of 16.60% and hazard ratios between 1.44 and 2.04, all p < 0.001), co-occurred more commonly, and formed a more interconnected network. Significant differences in incidence and co-occurrence were associated with sex, age, and illness severity. Besides the limitations inherent to EHR data, limitations of this study include that (i) the findings do not generalize to patients who have had COVID-19 but were not diagnosed, nor to patients who do not seek or receive medical attention when experiencing symptoms of long-COVID; (ii) the findings say nothing about the persistence of the clinical features; and (iii) the difference between cohorts might be affected by one cohort seeking or receiving more medical attention for their symptoms.
Conclusions: Long-COVID clinical features occurred and co-occurred frequently and showed some specificity to COVID-19, though they were also observed after influenza. Different long-COVID clinical profiles were observed based on demographics and illness severity.
- Quentin Dercon, Jennifer M. Nicholas, Sarah-Naomi James, Jonathan M. Schott, and Marcus Richards
Background: Grip strength is an indicator of physical function with potential predictive value for health in ageing populations. We assessed whether trends in grip strength from midlife predicted later-life brain health and cognition.
Methods: 446 participants in an ongoing British birth cohort study, the National Survey of Health and Development (NSHD), had their maximum grip strength measured at ages 53, 60-64, and 69, and subsequently underwent neuroimaging as part of a neuroscience sub-study, referred to as "Insight 46", at age 69-71. A group-based trajectory model identified latent groups of individuals in the whole NSHD cohort with below- or above-average grip strength over time, plus a reference group. Group assignment, plus standardised grip strength levels and change from midlife were each related to measures of whole-brain volume (WBV) and white matter hyperintensity volume (WMHV), plus several cognitive tests. Models were adjusted for sex, body size, head size (where appropriate), sociodemographics, and behavioural and vascular risk factors.
Results: Lower grip strength from midlife was associated with smaller WBV and lower matrix reasoning scores at age 69-71, with findings consistent between analysis of individual time points and analysis of trajectory groups. There was little evidence of an association between grip strength and other cognitive test scores. Although greater declines in grip strength showed a weak association with higher WMHV at age 69-71, trends in the opposite direction were seen at individual time points with higher grip strength at ages 60-64, and 69 associated with higher WMHV.
Conclusions: This study provides preliminary evidence that maximum grip strength may have value in predicting brain health. Future work should assess to what extent age-related declines in grip strength from midlife reflect concurrent changes in brain structure.